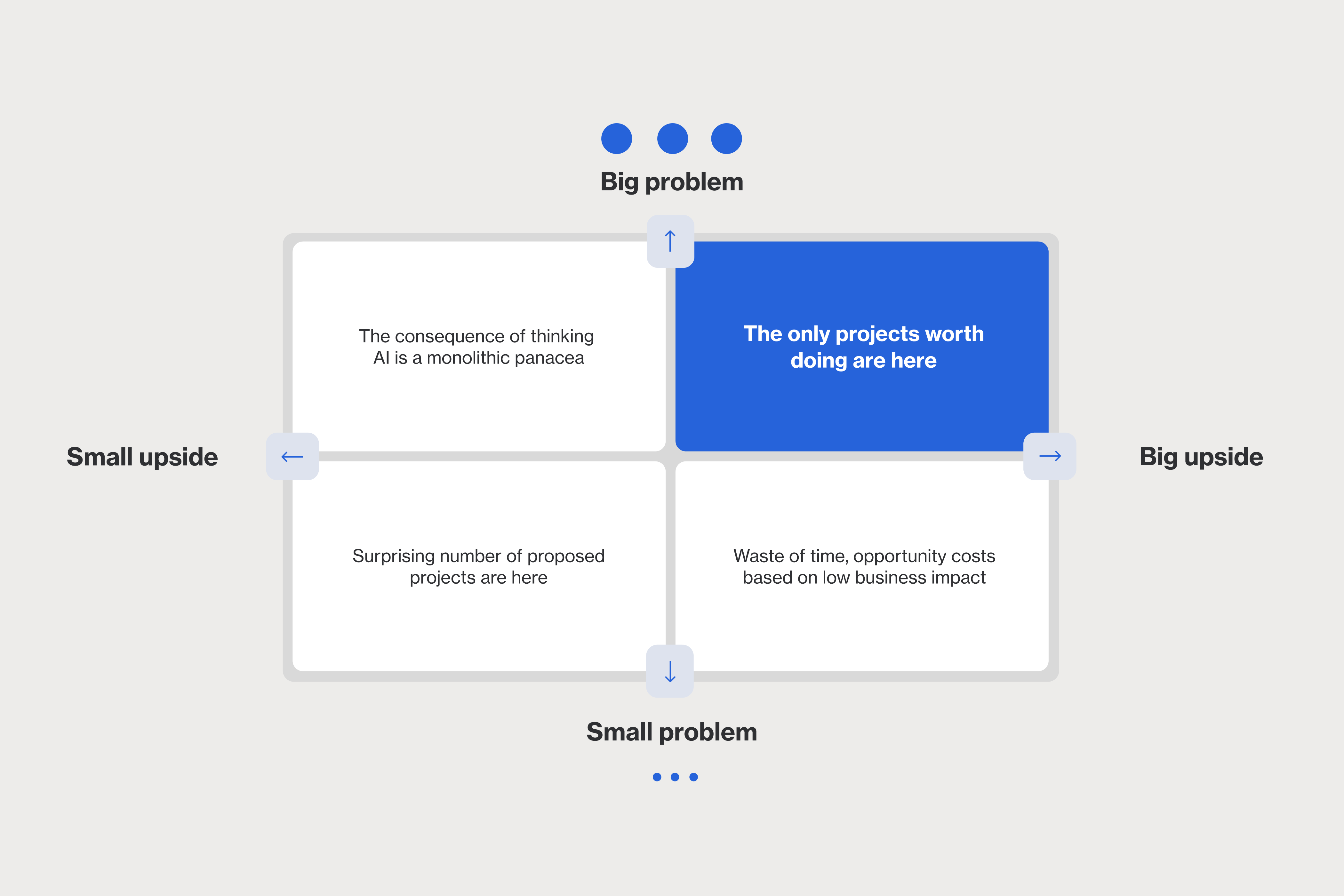
Companies and investors are both overestimating and underestimating the capabilities of generative AI
At NLX, we couldn’t agree more that the tangible results from generative AI have not lived up to the hype in the broader market, creating the so-called generative AI bubble.
Having said that, it’s wrong to conclude that the technology is not yet capable of delivering the business transformation that we imagined. The reality is that the market has both overestimated AND underestimated what generative AI can do. Here’s what I mean:
We overestimate what AI can do when we think it can solve any business problem with its human-like powers of deduction.
Despite what we see in the movies, AI is not sentient; it has no soul and no conscience. It cannot solve ambiguous, nuanced, and human problems. And even if generative AI were good at handling structured business processes, which it’s not, companies shouldn’t spend the money on it when much cheaper solutions are available.
Yet, companies wrongly believed that slapping generative AI solutions onto any myriad of problems would not only work from a technology perspective but be worth the money. Turns out that is hardly ever the case.
On the other hand, if the appropriate AI technology is applied to a properly sized and scoped problem, with clear goals and metrics set in advance, the latest generative AI applications can do MORE than we think possible.
AI technology is not monolithic. There are different types and levels that are good for different things with vastly different costs.
Further, AI is not a good solution for every problem. Some problems are better solved with other types of technology. Ironically, we often see companies trying to solve the easiest problems with AI, which is expensive and unnecessary, when they could be investing those resources in solving more complex problems with the potential for higher business impact.
Finally, even if AI is well-suited to solve a problem, don’t bother if it’s too small to make a material difference in the business. In other words, even if the tech works, it can still be a failure because the cost or effort isn’t worth the results.
OK, so we’re choosing a big problem. That means it will be even more visible if the AI project fails. CORRECT! How do you know if it’s appropriate for AI to solve and what kind of AI to choose?
First, identify the metrics that need to move and by how much. Next, dissect the problem piece by piece and determine the necessary outcomes to move a target metric. Then, determine if AI can move any key metric, and if so, what specific technology is most likely to work. Part of that decision-making process is determining the tolerance for risk at each step. Generative AI, like all forms of AI, is rooted in statistics, which means probabilities, which means inherent imperfection.
For example, a hospital system might use a combination of AI technologies to triage patients, such as determining what location a patient should visit or what specialty department they should see. Interestingly, with NLX, AI could perform the triage, recommend open appointment times, schedule the appointment, check whether the specialist was in the patient’s insurance network, and estimate charges beyond the copay. That’s an example of AI going beyond what we might imagine is possible today.
The hospital system might determine that the thousands of patients trying to navigate their system, book appointments, and understand their insurance costs is a big enough problem to solve. They know the human labor costs to serve the patients who stick it out through the process and the lost revenue from patients who drop because it’s too many steps to figure everything out.
Great. Then, they break down the steps in the process and determine where traditional AI, like natural language processing (NLP), is sufficient and when generative AI applications are needed. They estimate the cost and determine if the ROI is there.
An ill-advised use of AI technology would be determining medication doses for patients. Even if the AI were more efficient, there is no room for error. And would the ROI actually be material to the hospital, given the risk? Probably not.
This might seem overly obvious and simplistic, but believe me, examples like this created the generative AI bubble. Companies using LLMs are investing hundreds of millions of dollars in poorly conceived and executed projects on the customer side. At the end of the day, much of the narrative about the disappointments of generative AI is true. CIOs are being inundated with project ideas and requests while dealing with tech debt and dated systems that are already limping along. It takes time for any new technology to transform billion-dollar organizations. Generative AI has massive potential and will eventually deliver value to the economy. Companies are already beginning to adjust their approach, focusing on large-scale problems that AI is well-suited to solve and deploying the right AI technologies in the right places.
What’s untrue is that generative AI is not yet powerful or advanced enough to have a transformative impact on business and the global economy. Indeed, it is. But it’s still only a tool, not a sentient being. And like any tool, no matter how powerful, the success or failure it brings, the good or evil it does, all depend on how it’s applied by the humans who use it.